There are plenty of places for life to hide. Even on our blue planet, where we know there is abundant life, it is sometimes difficult to predict all the different environments it might crop up in. Exploring worlds other than our own for life would make it exponentially more difficult to detect it because, realistically, we don’t really know what we’re looking for. But life will probably present itself with some sort of pattern. And there is one new technology that is exceptional at detecting patterns: machine learning. Researchers at the SETI Institute have started working on a machine-learning-based AI system that will do just that.
Continue reading “Finding Life in the Solar System Means Crunching a Lot of Data. The Perfect Job for Machine Learning”Machine Learning is a Powerful Tool When Searching for Exoplanets
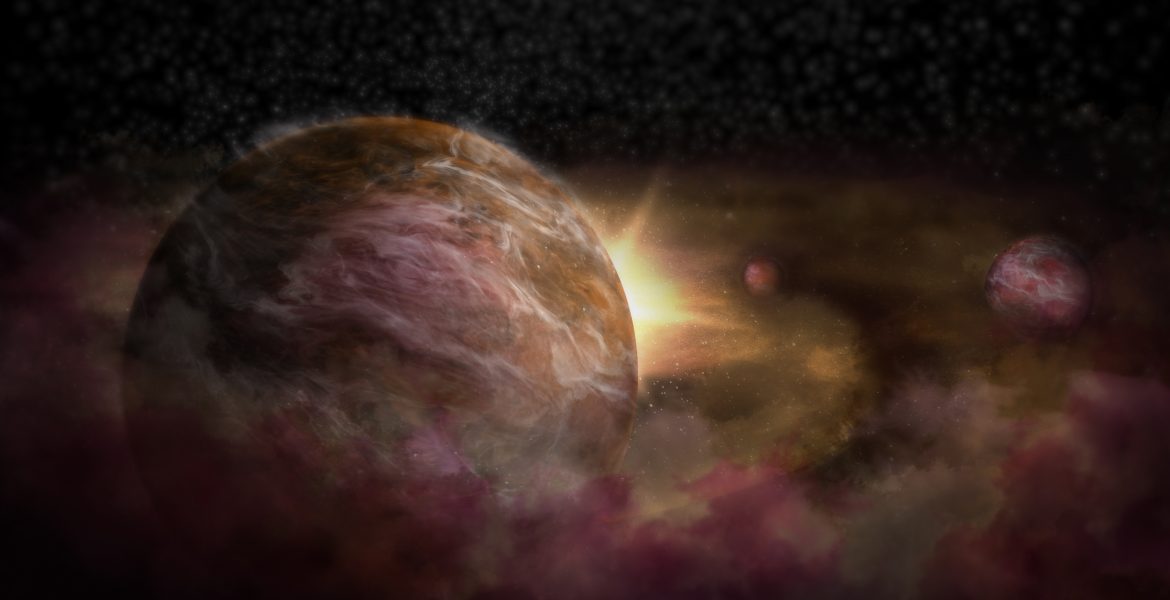
Astronomy has entered the era of big data, where astronomers find themselves inundated with information thanks to cutting-edge instruments and data-sharing techniques. Facilities like the Vera Rubin Observatory (VRO) are collecting about 20 terabytes (TB) of data on a daily basis. Others, like the Thirty-Meter Telescope (TMT), are expected to gather up to 90 TB once operational. As a result, astronomers are dealing with 100 to 200 Petabytes of data every year, and astronomy is expected to reach the “exabyte era” before long.
In response, observatories have been crowdsourcing solutions and making their data open-access so citizen scientists can assist with the time-consuming analysis process. In addition, astronomers have been increasingly turning to machine learning algorithms to help them identify objects of interest (OI) in the Universe. In a recent study, a team led by the University of Georgia revealed how artificial intelligence could distinguish between false positives and exoplanet candidates simultaneously, making the job of exoplanet hunters that much easier.
Continue reading “Machine Learning is a Powerful Tool When Searching for Exoplanets”More Data and Machine Learning has Kicked SETI Into High Gear
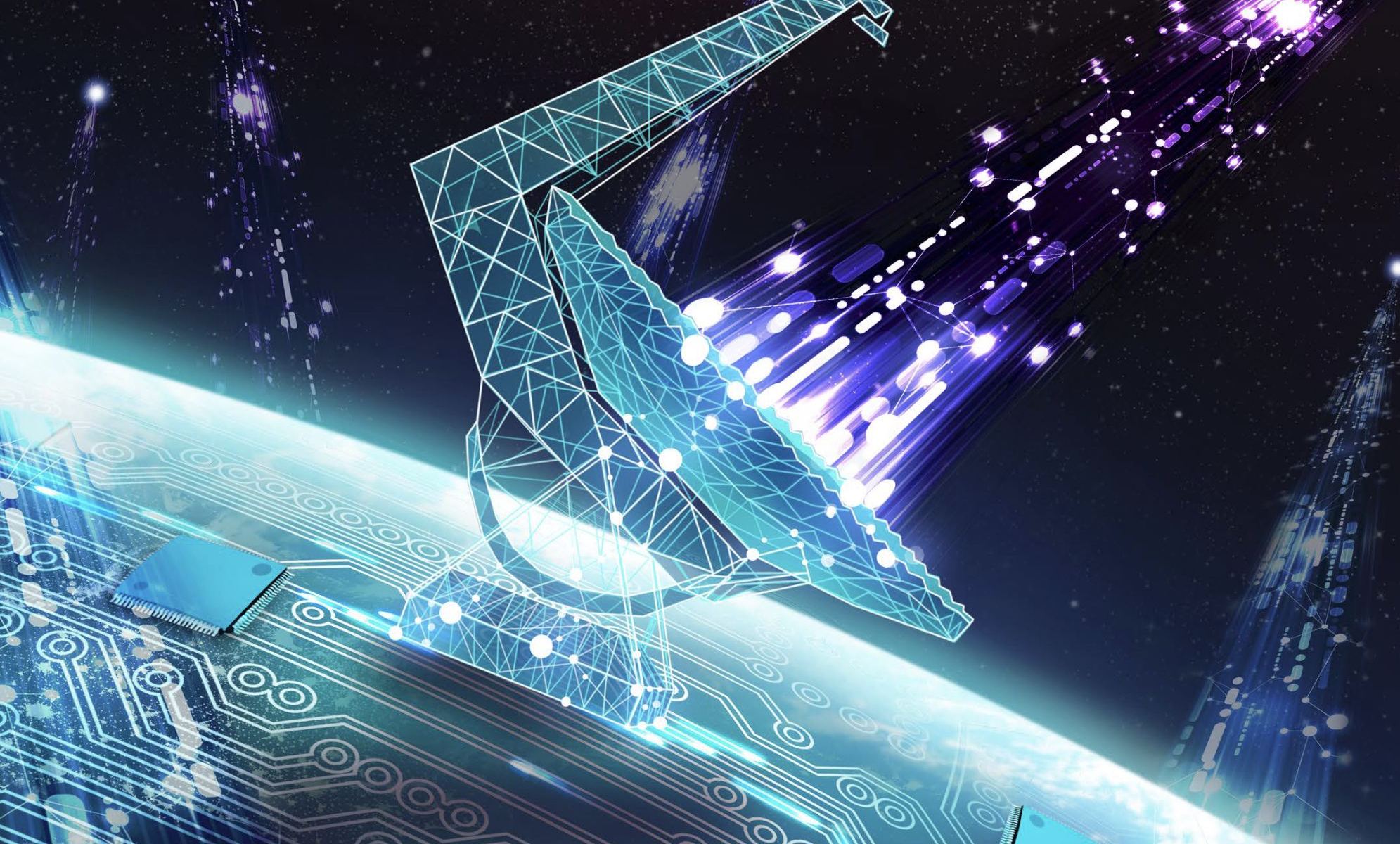
For over sixty years, astronomers and astrophysicists have been engaged in the Search for Extraterrestrial Intelligence (SETI). This consists of listening to other star systems for signs of technological activity (or “technosignatures), such as radio transmissions. This first attempt was in 1960, known as Project Ozma, where famed SETI researcher Dr. Frank Drake (father of the Drake Equation) and his colleagues used the radio telescope at the Green Bank Observatory in West Virginia to conduct a radio survey of Tau Ceti and Epsilon Eridani.
Since then, the vast majority of SETI surveys have similarly looked for narrowband radio signals since they are very good at propagating through interstellar space. However, the biggest challenge has always been how to filter out radio transmissions on Earth – aka. radio frequency interference (RFI). In a recent study, an international team led by the Dunlap Institute for Astronomy and Astrophysics (DIAA) applied a new deep-learning algorithm to data collected by the Green Bank Telescope (GBT), which revealed eight promising signals that will be of interest to SETI initiatives like Breakthrough Listen.
Continue reading “More Data and Machine Learning has Kicked SETI Into High Gear”Not Just Stars. Gaia Mapped a Diverse and Shifting Universe of Variable Objects
We’ve reported on Gaia’s incredible data-collection abilities in the past. Recently, it released DR3, its latest data set, with over 1.8 billion objects in it. That’s a lot of data to sift through, and one of the most effective ways to do so is through machine learning. A group of researchers did just that by using a supervised learning algorithm to classify a particular type of object found in the data set. The result is one of the world’s most comprehensive catalogs of the type of astronomical object known as variables.
Continue reading “Not Just Stars. Gaia Mapped a Diverse and Shifting Universe of Variable Objects”A Computer Algorithm is 88% Accurate in Finding Gravitational Lenses
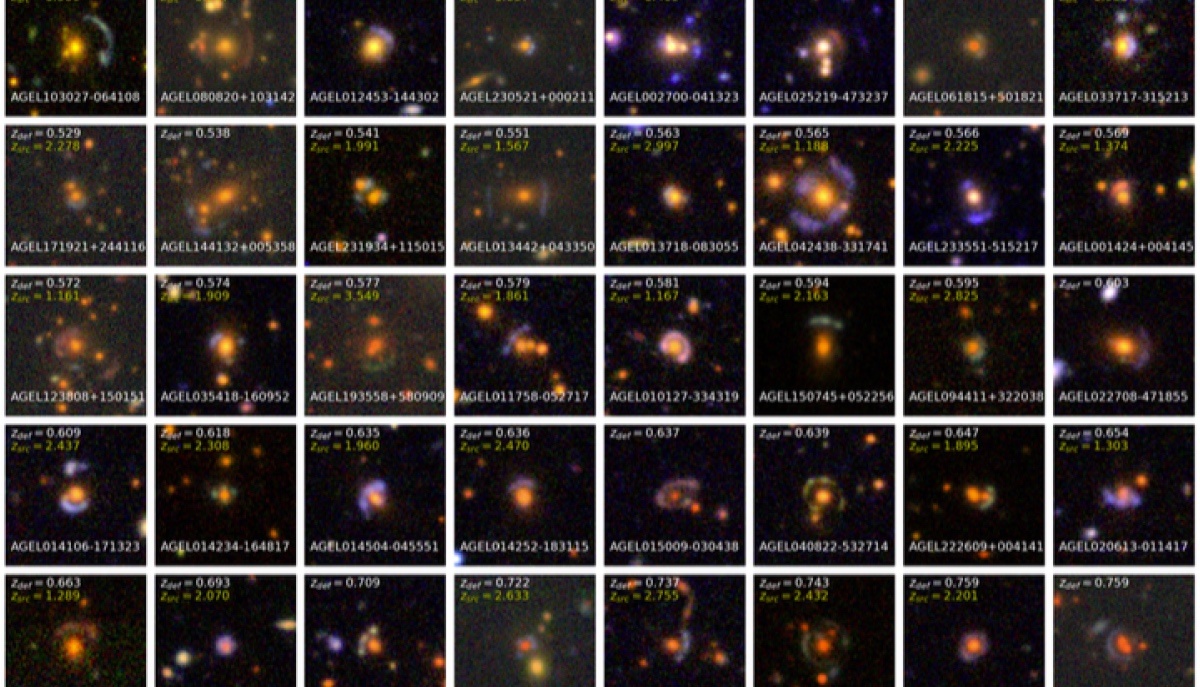
Astronomers have been assessing a new machine learning algorithm to determine how reliable it is for finding gravitational lenses hidden in images from all sky surveys. This type of AI was used to find about 5,000 potential gravitational lenses, which needed to be confirmed. Using spectroscopy for confirmation, the international team has now determined the technique has a whopping 88% success rate, which means this new tool could be used to find thousands more of these magical quirks of physics.
Continue reading “A Computer Algorithm is 88% Accurate in Finding Gravitational Lenses”If Aliens Were Sending us Signals, This is What They Might Look Like
For over sixty years, scientists have been searching the cosmos for possible signs of radio transmission that would indicate the existence of extraterrestrial intelligence (ETI). In that time, the technology and methods have matured considerably, but the greatest challenges remain. In addition to having never detected a radio signal of extraterrestrial origin, there is a wide range of possible forms that such a broadcast could take.
In short, SETI researchers must assume what a signal would look like, but without the benefit of any known examples. Recently, an international team led by the University of California Berkeley and the SETI Institute developed a new machine learning tool that simulates what a message from extraterrestrial intelligence (ETI) might look like. It’s known as Setigen, an open-source library that could be a game-changer for future SETI research!
Continue reading “If Aliens Were Sending us Signals, This is What They Might Look Like”Machine Learning Will be one of the Best Ways to Identify Habitable Exoplanets
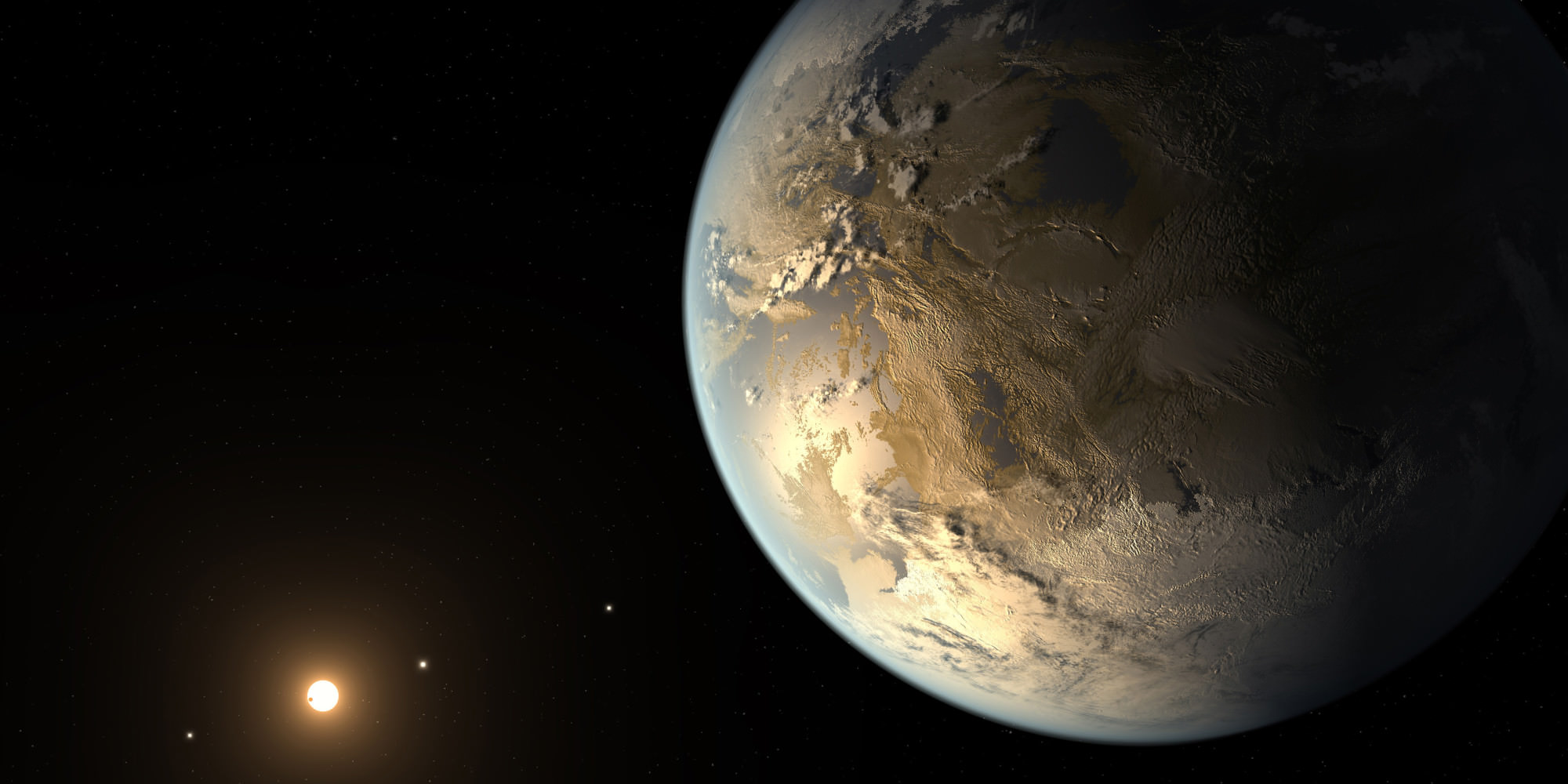
The field of extrasolar planet studies is undergoing a seismic shift. To date, 4,940 exoplanets have been confirmed in 3,711 planetary systems, with another 8,709 candidates awaiting confirmation. With so many planets available for study and improvements in telescope sensitivity and data analysis, the focus is transitioning from discovery to characterization. Instead of simply looking for more planets, astrobiologists will examine “potentially-habitable” worlds for potential “biosignatures.”
This refers to the chemical signatures associated with life and biological processes, one of the most important of which is water. As the only known solvent that life (as we know it) cannot exist, water is considered the divining rod for finding life. In a recent study, astrophysicists Dang Pham and Lisa Kaltenegger explain how future surveys (when combined with machine learning) could discern the presence of water, snow, and clouds on distant exoplanets.
Continue reading “Machine Learning Will be one of the Best Ways to Identify Habitable Exoplanets”A Machine-Learning Algorithm Just Found 301 Additional Planets in Kepler Data
Looking to the future, astronomers are excited to see how machine learning – aka. deep learning and artificial intelligence (AI) – will enhance surveys. One field that is already benefitting in the search for extrasolar planets, where researchers rely on machine-learning algorithms to distinguish between faint signals and background noise. As this field continues to transition from discovery to characterization, the role of machine intelligence is likely to become even more critical.
Take the Kepler Space Telescope, which accounted for 2879 confirmed discoveries (out of the 4,575 exoplanets discovered made to date) during its nearly ten years of service. After examining the data collected by Kepler using a new deep-learning neural network called ExoMiner, a research team at NASA’s Ames Research Center was able to detect 301 more planetary signals and add them to the growing census of exoplanets.
Continue reading “A Machine-Learning Algorithm Just Found 301 Additional Planets in Kepler Data”Researchers Have Taught a Drone to Recognize and Hunt Down Meteorites Autonomously
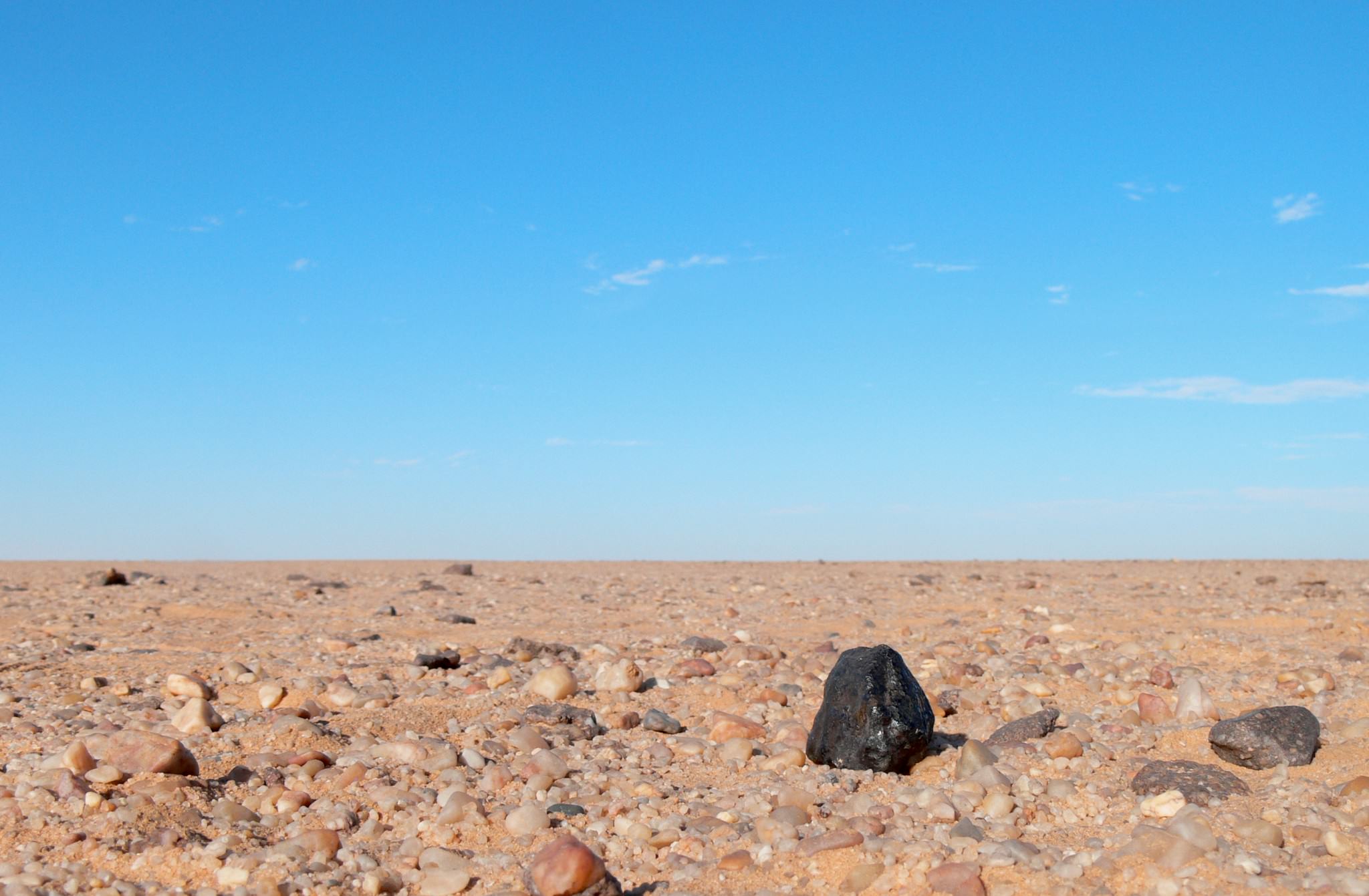
Planetary scientists estimate that each year, about 500 meteorites survive the fiery trip through Earth’s atmosphere and fall to our planet’s surface. Most are quite small, and less than 2% of them are ever recovered. While the majority of rocks from space may not be recoverable due to ending up in oceans or remote, inaccessible areas, other meteorite falls are just not witnessed or known about.
But new technology has upped the number known falls in recent years. Doppler radar has detected meteorite falls, as well as all-sky camera networks specifically on the lookout for meteors. Additionally, increased use of dashcams and security cameras have allowed for more serendipitous sightings and data on fireballs and potential meteorite falls.
A team of researchers is now taking advantage of additional technology advances by testing out drones and machine learning for automated searches for small meteorites. The drones are programmed to fly a grid search pattern in a projected ‘strewn field’ for a recent meteorite fall, taking systematic pictures of the ground over a large survey area. Artificial intelligence is then used to search through the pictures to identify potential meteorites.
Continue reading “Researchers Have Taught a Drone to Recognize and Hunt Down Meteorites Autonomously”The Most Comprehensive 3D Map of Galaxies Has Been Released
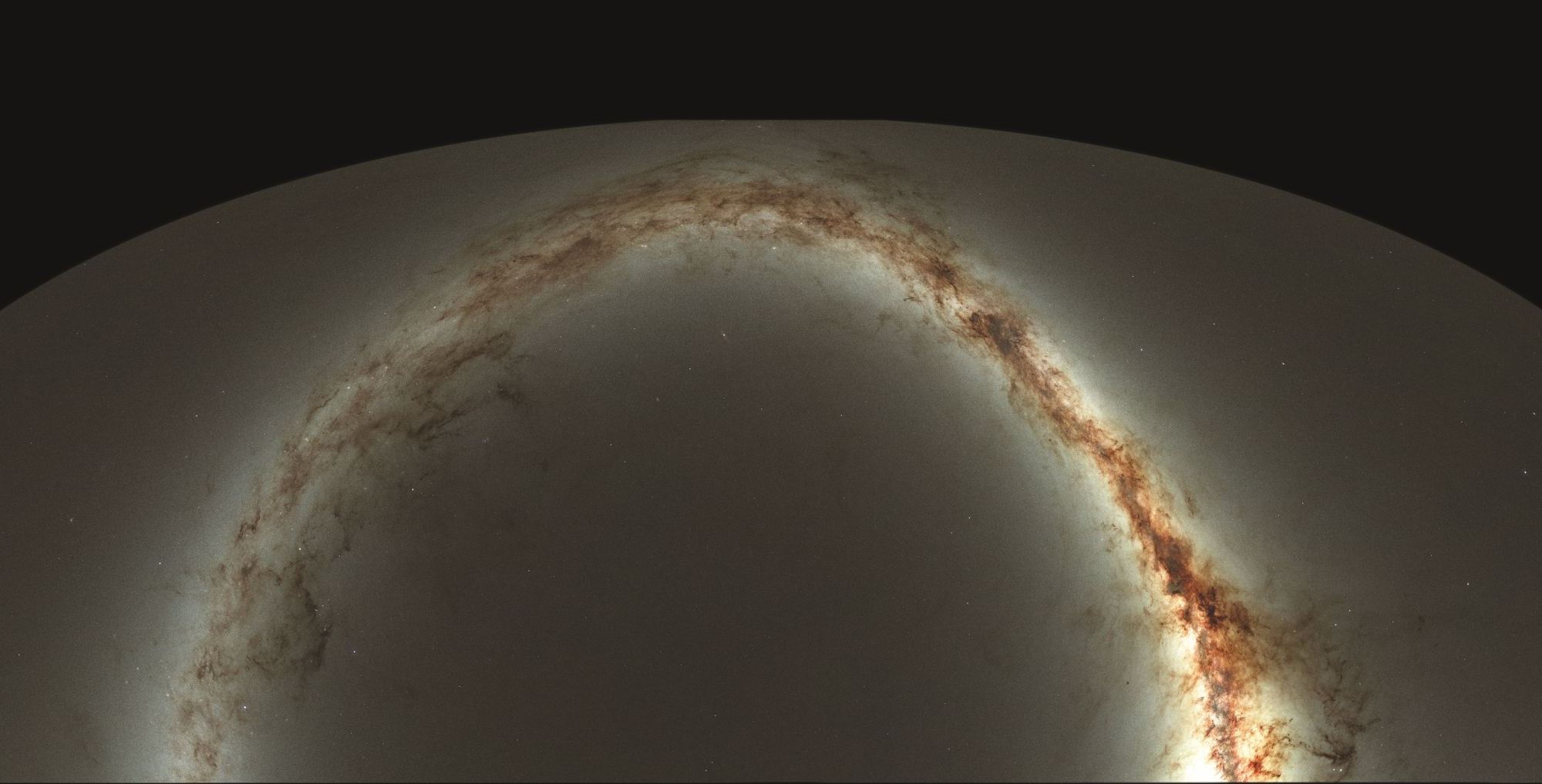
Atop the summit of Haleakala on the Hawaiian island of Maui sits the Panoramic Survey Telescope and Rapid Response System, or Pan-STARRS1 (PS1). As part of the Haleakala Observatory overseen by the University of Hawaii, Pan-STARRS1 relies on a system of cameras, telescopes, and a computing facility to conduct an optical imaging survey of the sky, as well as astrometry and photometry of know objects.
In 2018, the University of Hawaii at Manoa’s Institute for Astronomy (IfA) released the PS1 3pi survey, the world’s largest digital sky survey that spanned three-quarters of the sky and encompassed 3 billion objects. And now, a team of astronomers from the IfA have used this data to create the Pan-STARRS1 Source Types and Redshifts with Machine Learning (PS1-STRM), the world’s largest three-dimensional astronomical catalog.
Continue reading “The Most Comprehensive 3D Map of Galaxies Has Been Released”